Philipe Ambrozio Dias from the Marquette University’s Electrical and Computer Engineering Department in Milwaukee, USA presented a webinar on Tuesday, December 11, 2018, 12:30pm.
A video of the webinar is available at this link [115 MB] as well as on YouTube.
Talk details
Title: Towards automated bloom intensity estimation: (un)supervised learning, datasets and annotation tool for fruit flower segmentation.
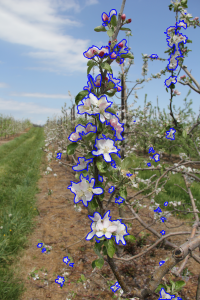
Labeling of apple flowers (outlined in blue) using CNNs, work of Philipe Dias and co-authors. Image courtesy Philipe Dias.
Abstract: Bloom intensity estimation provides early fruit yield estimation and is crucial to guide processes such as pruning and thinning, which directly impact fruit load and quality. Yet, human visual inspection is still the dominant approach for bloom intensity estimation in orchards, a technique time-consuming and prone to errors. In this presentation, we will be highlighting our recent progresses towards automated and more accurate methods for estimating bloom intensity. By means of finetuning, we have been exploiting convolutional neural networks (CNNs) for multi-species fruit flower detection. As modern CNNs tend to output rather coarse masks for segmentation tasks, we have also developed an unsupervised module for segmentation refinement. Since there is a lack of datasets for fruit flower detection, we annotated images of multiple species of fruit flowers and made them publicly available. To obtain such pixel-accurate annotation masks in a timely manner, we have been developing an open-source annotation tool that will also benefit the community.
Supplementary/Reading materials
Dias, P.A., Tabb, A. Medeiros, H. “Multispecies Fruit Flower Detection Using a Refined Semantic Segmentation Network” IEEE Robotics and Automation Letters, vol. 3, no. 4, 2018.
Publisher link, Open access: COVISS
Companion dataset:
Dias,P.A., Tabb, A., Medeiros, H. (2018). Data from: Multi-species fruit flower detection using a refined semantic segmentation network. Ag Data Commons. http://dx.doi.org/10.15482/USDA.ADC/1423466
Dias, P.A., Tabb, A., Medeiros H.“Apple flower detection using deep convolutional networks,” Computers in Industry, vol. 99, 2018.
Publisher link, Open access: arXiv
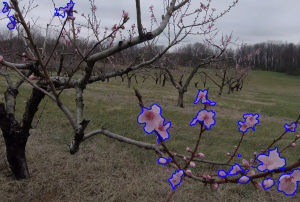
Labeling of peach flowers (outlined in blue) using CNNs, work of Philipe Dias and co-authors. Image courtesy Philipe Dias.
Dias, P. A.; Medeiros, H. “Semantic Segmentation Refinement by Monte Carlo Region Growing of High Confidence Detections” Asian Conference on Computer VIsion (ACCV), 2018. [to appear]
Dias, P. A.; Shen, Z.; Tabb, A.; Medeiros, H. “FreeLabel: A Publicly Available Annotation Tool based on Freehand Traces” Winter Conference on Applications of Computer Vision (WACV), 2019.
Biographical information
Philipe Ambrozio Dias is a PhD student in Electrical and Computer Engineering at Marquette University. At the COVISS Laboratory, he works with Dr. Henry Medeiros on the development of novel machine learning algorithms for image and video understanding tasks. Philipe has been exploring topics such as convolutional neural networks, unsupervised clustering for segmentation refinement, and transfer learning for applications in agricultural and health-care scenarios.
Philipe received his Bachelor’s degree in Electrical Engineering and M.S. in Electrical and Computer Engineering from the Federal University of Technology, Brazil. He also holds a M.S. degree in Information Technology from the Hochschule Mannheim in Germany.